The limits of Artificial Intelligence and why it matters
23 May 2023
The public release of ChatGPT has sparked debate about how Artificial Intelligence (AI) will reshape society. Craig Webster says we need to take seriously the foundational limitations inherent in the technology.
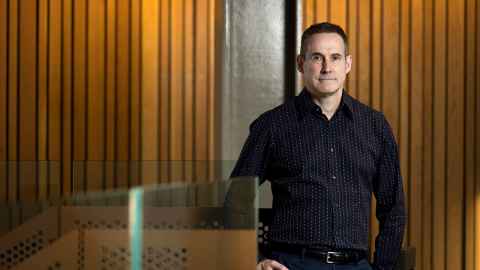
The rise of Artificial Intelligence (AI) may seem unstoppable. In the guise of ChatGPT and its upgrades and plugins, it took only 120 days from public launch to reach 1 billion users and at their behest, many billions of words from bad haiku to exemplary law exam answers have been generated. Other AIs such as Dall-E-2 and Midjourney have done similar things for images, creating re-imagined Rembrandts to deep fake celebrity videos.
Associate Professor Craig Webster says, “These new kinds of technologies are irresistible. There’s no going back and the problem with that is that many of us don’t really understanding their limitations.”
Webster is Associate Professor in the Centre for Medical and Health Science Education at the Faculty of Medical and Health Sciences at Waipapa Taumata Rau, University of Auckland. His interest in AI stemmed initially from its application in clinical settings. When it comes to pattern recognition, a properly trained AI is going to spot things a highly experienced human doctor might miss.
In the contest between human and machine, in areas like radiology, the machine has often proven to be better. “The AIs are very good at spotting signs and patterns of pathogenesis that need to be dealt with.”
The bigger question for Webster is when and under what circumstances we need a human in the loop. “A human doctor understands what it means for the patient to have lung cancer. The AI has no worldly understanding of the consequences of the tasks it performs.”
The key notion is ‘worldly’. AI is very new, Webster says. Human intelligence is the result of billions of years of evolution in the real world. In the deep past when the first single cells appeared, the cells that moved away from ‘noxious’ stimuli survived, the ones that failed to did not. Over endless millennia, evolution did its work. Human intelligence is the legacy of those billions of years of evolutionary pressure.
AI is a zombie intelligence. There’s nobody home, or anybody home to explain its actions or say how it arrived at its conclusion.
Webster: “That’s the important distinction, human intelligence possesses awareness of our own state and the world around us. This is very different to what deep learning Artificial Intelligence does.”
Through deep learning, AIs are trained on vast volumes of data in a virtual environment, and essentially become complex mathematical filters without any understanding of what passes through the filter. This situation was first explored by the philosopher John Searle in the 1980s in what he called the Chinese Room Argument.
In this thought experiment, an English speaker who does not understand Chinese is in a room with an input and an output slot, and a thick rule book written in English. A message in Chinese arrives through the input slot and the English speaker uses the rule book to match symbols and compose a reply in Chinese, which is then sent through the output slot. Despite the English speaker in the room having no understanding of Chinese, the Chinese speaker outside the room who is receiving replies feels they are communicating with a person fluent in Chinese.
While many technologists dismiss this argument as philosophical nonsense, Webster says that “All narrow AIs today are Chinese rooms. There’s nothing inside the room that understands what it’s doing.”
Webster says: “You could say that it doesn’t matter what happens inside the room as long as it gives the right answers and that’s a position a lot of computer scientists take. As long as the system responds in an intelligent way it doesn’t matter what goes on inside the system.”
But it’s not intelligence as we know it in the human sense. Rather says Webster it is a zombie intelligence, “The lights are on, but there’s nobody home, and the AI can’t explain its actions or say how it arrived at its conclusion.”
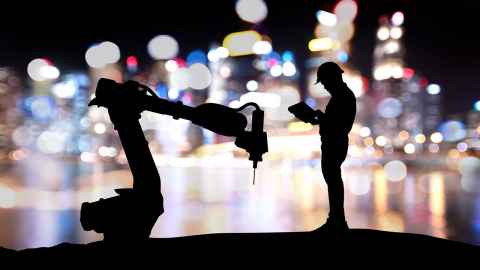
When it comes to writing, ChatGPT appears to do whatever is asked of it, whether a haiku or a university level essay. As Webster notes, it remains fallible, likely to make things up when it comes across a gap in its deep learning.
For example, if you ask ChatGPT: Who has the world record for crossing the English Channel entirely on foot? Humans cannot walk on water, and you can’t walk through the Channel Tunnel, so the question is actually nonsense, but ChatGPT doesn’t detect this, and hallucinates an answer: “The world record for the fastest crossing of the English Channel entirely on foot is six hours and 57 minutes, set by Yannick Bourseaux of France on September 7, 2012.”
Bourseaux is a real person, a paralympic athlete competing in the biathlon and cross-country skiing, but the rest of the information is entirely fabricated. Humans have been known to make things up as well, though usually with intent, but in this case it’s a glitch in the system.
This becomes less of a philosophical and ethical debate when AIs are deployed in the real world. They drive cars, respond to our internet searches, plot our destinations and look for cancerous growths. What concerns Webster are bizarre or catastrophic failures that can have real world consequences.
The best-known example is the autopilot self-driving system in Tesla cars. Fortune magazine reports that in a four-month period in 2022, 11 people were killed in crashes involving vehicles with automated driving systems, with ten of the deaths involving people driving Teslas.
Tesla founder Elon Musk has claimed that based on the rate of crashes and total distance driven, the Tesla automated systems were safer than a human driver, a claim often challenged by road safety experts.
Webster says, “The total crash rate might be less than for other cars, but that doesn’t mean we should accept these crashes.”
In these cases, the AI has often acted in a way that no human would, for example driving the vehicle directly under a container truck. Webster’s argument is that humans share an innate ability to make sense of our three-dimensional world where we evolved, while AIs do not.
Webster cites the example of attempts to train AIs to recognise a stop sign. They appeared to master this readily. “However, if the sign was damaged or marked or slightly off plumb or had stickers on it, things that happen quite often in the real world, the AI often saw something entirely different rather than a stop sign.”
This illustrates what Webster considers the foundational issue with AI. “These failure modes are part of the alignment problem. How do we align AI goals with human goals? We don’t want AIs giving people the wrong information or making the wrong decision, but even more so we don’t want AIs in charge when there is any possibility of dangerous consequences of bizarre or catastrophic failures.”
Humans are best placed to make decisions about context in the real world, something which is particularly relevant in healthcare. “We have to remain the sense makers, because we actually understand the world and the consequences of decisions made in that world.”
Key terms in the Artificial Intelligence arena
- Generative AI: Technology that creates content, text, images, video, code, by identifying patterns in large volumes of data and then creates original material with similar characteristics. Chat GPT is a generative AI.
- Large language model: A neural network that learns skills, e.g. writing prose, computer code by analysing vast amounts of text from the Internet. It’s predictive text on steroids.
- Natural language processing: Techniques used by large language models to understand and generate human language. The techniques often rely on a combination of machine learning algorithms, statistical models and linguistic rules.
- Neural network: A mathematical system similar to the human brain that learns skills by finding patterns in data.
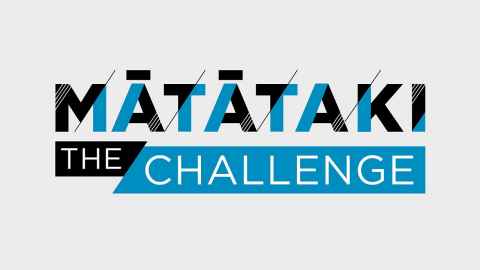
Writer: Gilbert Wong
Main image: Christopher Loufte
Mātātaki|The Challenge is a continuing series from Waipapa Taumata Rau, the University of Auckland, about how our researchers tackle some of the world's biggest challenges. Challenge articles are available for republication.
For re-publication requests: Gilbert Wong, Research Communications manager gilbert.wong@auckland.ac.nz