Automated pose estimation for prediction of cerebral palsy
10 December 2024
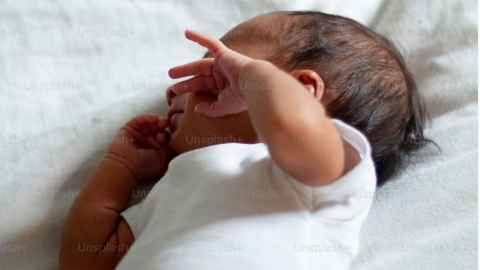
Cerebral palsy is the most common cause of child-onset physical disability in
New Zealand, and there’s no known cure, but Faculty of Science researchers believe that artificial intelligence can play a key role in supporting early identification of the condition – and help facilitate early intervention.
As a neuroscientist and senior lecturer in Exercise Science, Dr Angus McMorland heads a project funded by the Friedlander Foundation that is harnessing the power of AI to reveal the tell-tale signs of CP within the first few critical weeks after birth.
“Where you can make a meaningful difference for their whole life really has a maximum impact, and that’s what attracted me to it.”
It’s estimated that one baby is born with CP every three days in New Zealand, resulting in abnormal brain development and affecting functions like muscle control, posture and balance. Unfortunately for many youngsters, the more mild cases might not be diagnosed until around two years of age when the time-sensitive window for the optimised benefits of early intervention has passed.
“So then it becomes a much more physical condition rather than just a neurological one,” says Angus. “If we could intervene earlier on, then we can potentially reduce some of those consequences.”
Following the recent introduction of new best practice guidelines for the early prediction of CP, clinicians have begun using short video clips of babies younger than three months to complete a General Movement Assessment (GMA), which determines whether movement patterns are normal, abnormal or absent in newborns.
As Angus puts it, “We’ve been watching babies lying on their backs squirming around for thousands of years.”
However, the main focus of the GMA is on the ‘writhing’ movements that take place up to nine weeks of age and the ‘fidgety’ movements that occur up to 20 weeks.
“These movements wax and they wane in amplitude, and they show elegance and complexity and they’re smooth and fluid – all these sorts of things. So those are the hallmarks of healthy General Movements.”
Unfortunately, there are precious few trained clinicians in New Zealand, many of whom Angus says are conducting GMAs “on the side” in addition to their day jobs.
“There’s no way that the existing number of clinicians can do the number of tests that we would like the system to be doing.”
Which is where AI comes in. Using the video clips of 87 babies identified by Waikato Hospital as high-risk, researchers have been training what Angus describes as a “naïve” off-the-shelf AI motion-tracking platform called DeepLabCut™ to recognise infant body positions.
It’s been a painstaking process for research fellow Dr Hamid Abassi and PhD student Manpreet Kaur, involving the manual labelling of hundreds of video frames with wrist, elbow and shoulder movements – although Angus and his team have gone a step further by including rotational movements and some facial features that the AI platform can also recognise.
“They’re getting 98 percent accuracy in terms of where each of those markers is on the body now, so that’s working really well.”
The next phase in the creation of an automated GMA is to train a second AI system to determine whether movements are normal, abnormal or absent, which involves labelling movement patterns based on relevant clinical assessments.
“We think we’ve got some good ideas about how to do it really well, it’ll definitely work, and we think we can improve on some of the other approaches,” says Angus, referring to similar research being conducted elsewhere in the world.
The ultimate goal is to create a mobile app on a clinician’s phone or device which will automatically upload video content to the New Zealand eScience Infrastructure (NeSI) supercomputer which will process the images and send a determination back to the user.
Automating the process will hopefully remove some of the current time-consuming challenges involved in identifying high-risk babies, meaning that more babies will have the opportunity to be assessed.
“That’s one of the benefits of our system” says Angus, “identifying in a
much more specific way which babies are really going on to develop cerebral palsy will help with the development of these interventions – or the testing of them.”
Conventional interventions are generally based around increasing sensory feedback through play and time spent with parents, but testing these interventions has been difficult because of the challenges involved in identifying soon enough which babies will develop the condition.
Indeed, random samples of high-risk babies suggest that most wouldn’t be affected, which is why Angus says there needs to be better testing of current interventions. “Maybe there’s something else that we can do that’s even more targeted and going to have more of an effect.”
Looking ahead, he would like to develop AI systems that can track neurological outcomes at the age of two years when movement and cognitive and behavioural impairment may start to show – or not – although he admits that there’s likely to be some complexity involved in comparing video content and patient outcomes over a longer timeframe.
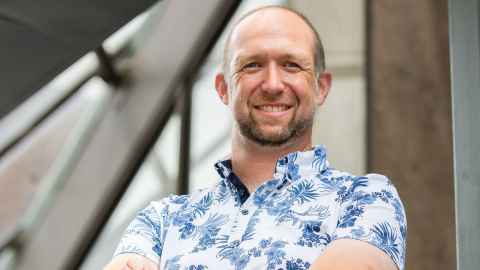
What we don’t know is how that impacts the networks of neurons and therefore the way that different parts of the brain are communicating with each other and how that impacts development to cause – ultimately – cerebral palsy.
“Because we’ve got these measurements of movements, we could start to improve upon what the clinicians can do. Is there something subtle in these movement patterns that tells us about this less severe condition at two years of age?”
Given that a child’s movements essentially provide a “window” into what’s going on in the brain, Angus also wants to investigate, as part of his blue-sky thinking as a neuroscientist, what changes have taken place at a neuron level – say from low oxygenation – to cause cerebral palsy.
“What we don’t know is how that impacts the networks of neurons and therefore the way that different parts of the brain are communicating with each other and how that impacts development to cause – ultimately – cerebral palsy.
Yet another long-term goal is to make the AI technology for CP diagnosis universally available, perhaps through Plunket, much in the same way that his son’s hearing difficulty was picked up by the nationwide programme to screen the hearing of newborns.
As he points out, the current practice is to look only at high-risk babies because that’s where the resources have to go.
But the reality is that of those diagnosed with CP, something like 45 percent had no appreciable medical history to suggest that they would develop the condition.
“The only way you could possibly pick them up is with a broad screen, and then you could intervene earlier than we do at the moment.”
Given the wide range of risk factors for CP, including infections, prematurity and complications at birth, Angus says the condition is “not going away.” And while the incidence in the Māori and Polynesian communities is equivalent to the wider population, “the consequences of that in terms of interacting with the health system are not necessarily equitable, so the outcomes are different.”
With that in mind, he would love to see the AI-generated diagnostic tool become widely available within the health sector, although it might require additional funding – perhaps from a not-for-profit organisation – to keep it going.
“My end goal is just to put it out there and make it available,” says Angus. “There’s no way that people should be paying for this privately, as far as I’m concerned.”
This story first appeared in InSCight 2024. Read more InScight stories.