Integrating AI and academia
10 December 2024
A look at the future of academia and research
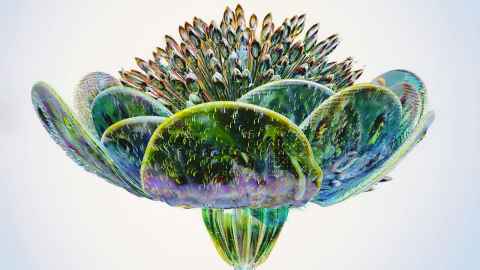
Professor Andrew Luxton-Reilly, Professor Mark Gahegan, and Associate Professor Paul Denny.
AI IS REDEFINING our society’s future, and we must adapt. The Faculty of Science at Waipapa Taumata Rau, University of Auckland, is well-positioned to embrace this challenge with the measured consideration it demands. With several thought leaders in the field to boast, the University is an established voice in the global discourse.
AI in education: a revolution in the classroom
Generative AI is a disruptive technology fundamentally changing the workforce and revolutionising our social structures. For education, it heralds the most significant democratisation of education since the printing press, at an unprecedented speed. With this great opportunity comes a significant challenge: how do we maintain the value of human-led education? When a student can learn effectively from an artificially intelligent tutor with human-like interactions that provide personalised, adaptive responses on demand, then what value does university education offer? In a future world where machines can complete intellectual tasks more effectively than graduates, what is the purpose of the University? In the short term, we are grappling with adjusting assessments, communicating expectations to students and upholding academic integrity. In the long term, we must consider how the role of universities is evolving and how teaching and learning will change as scientific knowledge is accessible to all and the artefacts produced by humans and AI models become increasingly indistinguishable.
One of the core functions of universities – credentialing – will likely remain a key value. However, with AI making independent learning more feasible, universities must ask: what value do we offer students if credentials are insufficient? The answer lies in fostering deep, relational and scientifically grounded learning experiences. To be human is to learn, and the sense of accomplishment and joy associated with mastering new knowledge and skills is deeply embedded in the human condition. Universities, at their best, facilitate the development of synergistic learning communities, where like-minded individuals gather in the same space, often leading to transformative connections that are hard to replicate elsewhere. Many great scientific and technological innovations started with people physically meeting at University, sharing ideas, and thinking similarly. Such communities accelerate learning and provide a platform for developing future core capabilities: discerning what is real, what is credible and what is not, how to work effectively with a range of AI tools, and making ethical decisions about how to interpret, evaluate, and use output from Gen AI. Students who master these skills will take a leading role in shaping our future.
Certainly, the widely discussed concerns surrounding AI – plagiarism, overreliance, and the erosion of independent thinking – are real. As a faculty, we are responsible for teaching students how to engage critically with AI tools. The future of education lies in striking a balance between preserving the uniquely human capacities that make learning a personal process and leveraging AI’s strengths. Several courses within the Faculty of Science have already begun to explore the use of AI-powered tools and feedback mechanisms for enhancing student learning. Such tools can provide personalised and timely feedback, and enable curiosity-driven learning in a way that is otherwise difficult to scale. Academic teaching staff remain essential for motivating students, fostering curiosity, and helping to develop a sense of community critical for collaborative learning and intellectual growth.
The Faculty of Science is world-leading in several aspects of the emerging use of AI in education, locally and internationally. Our staff have organised international events such as the “Generative AI in Education” workshop at NeurIPS (the world’s premiere AI conference) and developed impactful pedagogies and tools, for example, to train students in formulating effective prompts to guide AI models to solve computational tasks. Collectively, we must continue to explore and share efforts to embrace generative AI, to enhance learning and toequip students with essential future skills.
AI in research: a revolution in the laboratory
When computers first emerged in research (1950s-70s), they were used primarily to scale up analysis and modelling activities. Their impact was huge in some fields (such as physics, chemistry, and biology), but marginal in others: analysis and modellingis just one step on the research lifecycle, and not all fields needed this scaled capability.
This time round is different. As we begin to grasp what Gen AI can do or will do soon, it is clear the impact on research will be massive: every aspect of the research lifecycle will be affected.
Gen AI is already helping to augment human researchers, automating tasks such as reading and summarising the relevant literature, proposing new hypotheses, writing computer code and scripts, describing computational code, data and workflows in text and diagrams – in other words helping with many research tasks, including the authoring of scientific articles, along with further improving analysis and modelling. And soon, we can anticipate AI tools that can reliably make and explain new scientific discoveries across many research areas. We can also expect that the current Gen AI methods will improve rapidly as they are taught to avoid some of the errors and hallucinations they currently exhibit.
These developments may seem astonishing, but the foundations have been in place for some time. Gen AI was first developed in the 1960s, and language models have existed since the 1980s. Scaling these technologies up proved challenging, but once that problem was solved, it suddenly became possible to learn from extensive and broad data collections (such as the ‘common crawl’ of the whole Internet). The amazing, emergent behaviour we see in these Gen AI tools results from what Peter Norwig calls the “unreasonable effectiveness of data”– the remarkable ability of large data sets to produce highly accurate models, even when the algorithms used to analyse them are relatively simple.
How will researchers adapt to these challenges in the longer term? As we always have, hopefully, with a sense of wonder at the discoveries we can participate in and with the humility to recognise that non-human intelligence has many useful qualities we can learn from. Better science will improve our citizens’ lives and our planet’s health. Ultimately, this is more important than how research gets done.
This story first appeared in InSCight 2024. Read more InScight stories.