The complexities of artificial intelligence
10 December 2024
Professor Markus Luczak-Roesch is the Co-Director of Te Pūnaha Matatini, Aotearoa New Zealand's Centre of Research Excellence for Complex Systems, hosted by the University of Auckland.
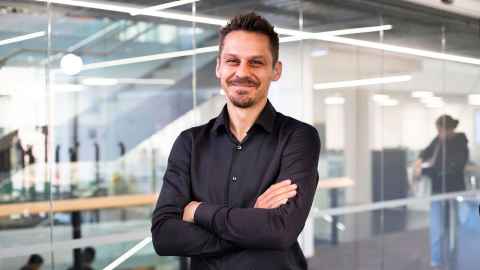
Just under two years ago, ChatGPT was launched. Within a few months, it had become the fastest-growing consumer application in history and is still one of the most visited websites on the Internet. In this remarkably short timeframe, many generative artificial intelligence (AI) tools like ChatGPT have become household names. They have been widely accepted in workplaces, and the government is actively working to increase their uptake by business.
AI refers to computer systems designed to perform tasks that typically require human intelligence, such as visual perception or language translation. The underlying principle of most current AI systems is to learn a “model” that allows them to computationally reproduce patterns found in large amounts of historic data. This can be as simple as a mathematical function or as complex as a network inspired by neuronal pathways in the human brain. These models can then be used to classify previously unknown data or predict the future trajectory of data.
Generative AI, the subset of AI that applications like ChatGPT belong to, focuses on creating content – such as text, images, or music – based on patterns learned from existing data. While generative AI excels at creative tasks and content generation, other AI forms are currently better suited for tasks like data analysis, autonomous navigation, or game-playing.
Predictions of the influence of AI range from utopian to dystopian. Some people expect AI to revolutionise human productivity and flourishing, while others have predicted the ultimate destruction of the human race. Thinking at both ends of this spectrum can be linear or reductionist– seeing AI as a mechanistic solution to mechanistic problems.
But the world is not mechanistic or linear, and there is a lot more that we need to understand about the complex ways in which AI will affect our lives and our planet.
Unveiling the complexity behind AI in our living rooms
In ‘Anatomy of an AI System’ (2018)**, Kate Crawford and Vladan Joler attempt to capture and map the system that lies behind a seemingly simple request to an Amazon Echo to turn the lights on in a hall. “In this fleeting moment of interaction, ”they say, “a vast matrix of capacities is invoked: interlaced chains of resource extraction, human labour and algorithmic processing across networks of mining, logistics, distribution, prediction and optimisation. The scale of this system is almost beyond human imagining. How can we begin to see it, to grasp its immensity and complexity as a connected form?”
There is a lot to consider. As Crawford and Joler have shown, “each small moment of convenience – be it answering a question, turning on a light, or playing a song – requires a vast planetary network, fuelled by the extraction of non-renewable materials, labour, and data.” And the evergrowing volume of requests to AI systems is collected, analysed, and retained to train the models that underpin these systems.
Consequently, promising positive growth through the deployment of AI falls into the trap of the traditional equilibrium model of economics, ignoring feedback and tradeoffs in the socio-economic fabric that may counterbalance gains.
Instead, we need to be thinking about the autonomous agents we are adding to our living rooms and what effects these agents will have on the complex systems they reside within.
We currently don't know how AI will behave in the world
Applications of AI will continue to grow. There are currently no limits to how many individual AI systems can be created and added to our world. And this comes at a great cost in terms of natural resources.
Altogether, there is little research so far about how different forms of AI – from the generative AI tools we know today to all sorts of other forms of autonomous agents and decision-making systems –will interact with and behave within the complex system of our social and natural environment. How will humans adapt when systems like this are brought into the world? And how will the AI systems adapt?
Studies have already shown that AI can plausibly add to the so-called market place of rationalisations and ideas. At times it does this so well that humans are more likely to adapt their beliefs when presented with AI-generated rationalisation compared with human ones, throwing up novel questions about how we model opinion dynamics and agency in opinion formation, for example.
Complexity science is now a recognised field that pushes the boundaries of our understanding of how to manage a world where everything is interdependent and where all our actions as individuals and collectives have hard-to-predict consequences.
We have the tools to find out
Luckily, complex systems researchers have been developing the perfect tools to study the behaviour of systems like artificial intelligence over many decades. The field of complex systems emerged in the mid-20th century, driven by the need to understand systems with numerous interacting components that exhibit unpredictable and emergent behaviours.
A series of fundamental ideas in mathematics, physics and the nascent field of computing led people to turn the traditional scientific paradigm – the one that puts disciplines like biology, economics or physics at the forefront of consideration – around and put the universal principles that can be found across all of those first. Some of these principles are scales, information, diversity, feedback, non-equilibrium, adaptation, self-organisation, memory, relationships, and non-linearity.
Complexity science is now a recognised field that pushes the boundaries of our understanding of how to manage a world where everything is interdependent and where all our actions as individuals and collectives have hard-to-predict consequences.
Recent research has suggested that the answers to questions about the effects of AI require network modelling and simulation – methods and tools central to complexity science. At Te Pūnaha Matatini, we go one step further and suggest that countries need reference models of synthetic populations that reflect the private and professional lives of their entire population and infrastructure to reliably simulate the effects when artificial agents are brought into the mix.
We need infrastructures to study AI before and while in use
A lot of claims have been made about how AI will affect our lives. With our current level of understanding, it is clear that many of these effects will be unexpected.
Before pharmaceuticals are released to the public, we conduct clinical trials on them and continue to track their effectiveness and adverse events while in broad use. We need to conduct trials with AI systems before these get rolled out and impact the lives of individuals and communities. We need infrastructures to track their workings, interactions, and adverse events while in use. With network modelling, simulation, and other methods from complexity science, we have the right tools for the job.
This story first appeared in InSCight 2024. Read more InScight stories.