Take 10 with... Joerg Wicker
Dr Joerg Wicker from the Department of Computer Science gives us 10 minutes of his time to discuss how machine learning methods can help us predict the environmental fate of pollutants.
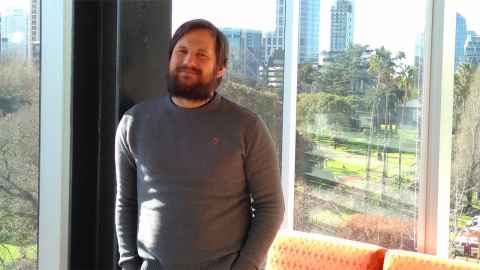
1. Describe your research topic to us in 10 words or less.
Machine learning methods to predict the environmental fate of pollutants.
2. Now explain it in everyday terms!
The chemical industry produces vast amount of chemicals that sooner or later end up in the environment, from agricultural chemicals, such as fertilizers or pesticides, to cosmetics and pharmaceuticals. These chemicals are not stable in the environment, but degrade to other chemicals. It is important to know the degradation processes and products of a chemical in the environment. A harmless chemical might degrade into something that is highly toxic and cause problems, while toxic chemicals such as pesticides might quickly degrade into something harmless and not cause any environmental issues.
Traditionally, experiments to measure degradation products are carried out in a chemistry lab and require expensive regular monitoring. In our research, we develop machine learning-based methods to predict the chemical reactions that happen in the environment. Machine Learning models are trained by learning from known biodegradation pathways of chemicals. The models can then predict potential reactions, based on reactions that were observed by similar compounds.
3. Describe some of your day-to-day research activities.
My main research activity is brainstorming with students and collaborators. My research is strongly interdisciplinary and my collaborators know far more about the application than me. While I depend on them to make sense out of the predictions of our models and identify problems, they rely on me to propose new computational approaches to solve complex problems.
4. What do you enjoy most about your research?
Computer science, and specifically machine learning, gives you the opportunity to collaborate with researchers from a wide range of disciplines and sciences, including sustainability. I enjoy diving into new topics and learning about other disciplines, and having the opportunity to see my research from a different perspective - through the eyes of my collaborators. This often leads to great discussions and interesting ideas for follow up research.
5. Tell us something that has surprised you in the course of your research.
A few years ago, we were contacted by an atmospheric chemist who put a device in a cinema to measure the concentration of small molecules in the air. Usually the concentrations of these molecules is measured outside, in the atmosphere. At first, measuring in a cinema seemed rather random, but it turned into an exciting project where we mapped the breath of crowds to emotional stimuli. It turns out we can predict what type of scene was running on screen based on the composition of the air in the cinema. For the first time, we were able to measure emotions of crowds based on their breath. It developed into a larger project in which we evaluate if humans communicate via smell.
6. How have you approached any challenges you’ve faced in your research?
I try to understand what is going on. I break everything down into smaller steps and make sense of all of them. I take whatever time is needed to understand the pieces, and don’t immediately discard them as a black box that 'should work'. Then it usually becomes clear where the issues are, and it is easier to fix several smaller problems than one huge road block.
7. What questions have emerged as a result?
It is not always easy to say if a model works well or not. Some traditional approaches of evaluating models are rather simple and rely on existing data sets. This approach can miss weaknesses of models, and simply using a calculated accuracy is not enough for models in critical environments. I frequently come across challenges when trying to prove to collaborators that a model works well and the predictions make sense. Domain experts usually want to understand why a specific prediction is made, not only that a model works in general.
As a result, my theoretical machine learning research went into the area of adversarial learning, where the goal is to actively find weaknesses of a model. By doing this, we not only rely on existing data sets, but create cases where something will go wrong. This is a fascinating area of research as it helps better understand how models work and why certain predictions are made. There are many unsolved questions on how to generate examples that make a model fail, or how it can be used to find better measures for model quality and reliability.
8. What kind of impact do you hope your research will have?
Ideally, the prediction of degradation of chemicals to prevent environmental pollutants from being released into the environment by early identification of potentially dangerous degradation products. The system we developed – enviPath – is already used by the chemical industry in the early assessment of their development pipeline. We also hope to help identify sources of pollution in the future and suggest mitigation strategies based on our models. This will help to reduce negative environmental impact.
The theoretical research in adversarial learning will help understand the risks and limitations of machine learning and provide a way to measure the quality of models before they are released and used in real life.
9. If you collaborate across the faculty or University, who do you work with and how does it benefit your research?
All my research relies on interdisciplinary collaboration, within the University and internationally. I collaborate with environmental researchers, chemists, other computer scientists, engineers, and medical scientists at the University of Auckland. I also have a long-running collaboration with the University of Waikato on machine learning topics. Internationally, I work with partners in the chemical industry, and atmospheric chemists at the Max Planck Society for Chemistry in Germany. My project on biodegradation is joint work with environmental chemists in Switzerland.
I enjoy these cross-disciplinary and international projects, and that computer science allows me to explore new areas of research. This gives me new perspectives that help me advance my research and give me new ideas. In my opinion, this external perspective can be beneficial in all research areas. The same way I get new ideas for machine learning algorithms, researchers from other disciplines benefit by approaching their challenges from a different direction, sometimes giving them a new way of asking questions and getting more out of their data. Frequently, there are ways to generate new knowledge from data that is non-trivial and an external perspective can help unlock this potential.
10. What one piece of advice would you give your younger, less experienced research self?
Discuss with others, share your ideas, and find collaborators who you enjoy working with. Science does not work if you sit in your office alone - it lives from discussions and arguments with others. It is very important to find collaborators that work well with you, and it is perfectly normal that not every potential collaborator fits with your way of working.