Take 10 with... Yalu Wen
Dr Yalu Wen, lecturer in the Department of Statistics, gives us 10 minutes of her time to discuss big genetic data, and their impact on our understanding of disease.
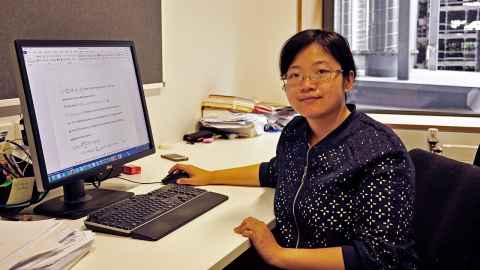
1. Describe your research topic to us ten words or less.
My research interest is primarily in statistical genetics (that is, statistical models in human genetics).
2. Now describe it in ‘layman’s’ terms!
I’m developing statistical methods to analyse high-dimensional genetic data. Big genetic data, with their potential to ascertain valued insights for the underlying disease process, have recently attracted substantial interest. They have the great potential to facilitate precision medicine. However, how to efficiently extract information from these big data is analytically challenging.
3. What are some of the day-to-day research activities you carry out?
My research is usually motivated by some real-world problems (for example, one of my collaborators wants to find genetic regions that are associated with lung cancer using the data he collected).
I first try to understand the complex nature of the high-dimensional datasets, and then develop statistical models to describe the data. I use simulation studies to thoroughly investigate the performance of my method, and then apply my method to the real datasets to see if it can help better address the original biological or medical questions.
4. What do you enjoy most about your research?
Usually in the course of developing new analytical methods for high-dimensional datasets, I’ll find other challenging problems that need to be solved. I really enjoy this problem-finding – and problem-solving – process.
5. Tell us about something that has surprised you or amused you in the course of your research.
When I did my PhD, I developed a single array approach to get the genotype calling.
Originally the method took about four hours to get the results, and later I realised that the program can be optimised to reduce the computational cost. I re-wrote the program, and it now takes about only two minutes!
I was surprised that a simple modification in the program can substantially improve the performance of a method. Given this experience, when I develop method, I always try to figure out if there’s another, faster way to implement it.
6. How have you approached any challenges you’ve faced in your research?
As a researcher, I face challenges every day. Some are easy to solve, while others are very hard.
When the problem is hard to solve, I usually try to cut it into pieces and then solve it piece by piece. I understand that my solution is not the best to many of the problems.
However, as long as my method is better than the existing methods, I can make some progress and then try to further improve it if I can.
7. What questions have emerged as a result?
I recently developed an analytical method to build disease risk prediction models using whole-genome DNA sequencing data.
When I finished this project, I realised that the method could be improved to increase its prediction accuracy. For example, the variable selection algorithm could be further improved by incorporating screening algorithms.
How to incorporate and develop the screening rules becomes a new question that I need to solve!
8. What kind of impact do you hope your research will have?
I hope my research could help biological and medical scientists to better understand disease mechanisms and predict disease risk accurately. Ultimately, I hope my research could facilitate precision medicine, and the process of delivering the right treatment to the right person.
9. If you collaborate across the faculty or University, or even outside the University, who do you work with and how does it benefit your research?
I collaborate extensively with biological and medical scientists. Although I mainly focus on developing analytical methods, the understanding of the disease mechanisms and the nature of the datasets is extremely important for model building and validating process.
If the model is to be practically useful, the input from biological and medical scientists is essential because their advice can help to assess the clinical utility of the methods.
10. What one piece of advice would you give your younger, less experienced research self?
Whenever you hit a bottleneck in your research, never give up!