How to make generative AI greener
Breaking down the carbon footprint lifecycles of machine learning models to identify methods for greener generative AI.
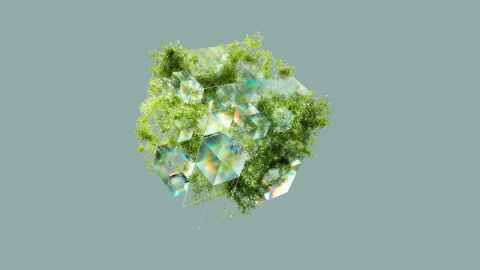
Article originally appeared on Harvard Business Review, July 20, 2023
By Ajay Kumar and Tom Davenport Harvard Business Review, July 20, 2023
What/Focus
Generative AI tools such as ChatGPT require large volumes of data and almost all are generated by “hyperscale” cloud providers using thousands of servers, which produce major carbon footprints through their water and energy use. By breaking down the carbon footprint lifecycles of machine learning models, the authors identify ways to make generative AI greener.
How (details/methods)
The three aspects of generative AI that use energy and produce emissions are training the models, running inference once the models are deployed, and producing the required computing hardware and cloud data centre capabilities. Training models is the most energy-intensive component of generative AI. However, while sometimes models are trained only once, they are then deployed to the cloud for use by millions for inference. In fact, 80–90% of the energy cost of neural networks lies in ongoing inference processing after a model has been trained. The third aspect is the cost of manufacturing the computers used to run AI software and the complex and powerful GPU chips and servers used to run AI models.
The following eight tips address different aspects of generative AI’s carbon footprint.
First, given the enormous amount of energy involved in generating models, it is better to use existing large generative language and image models rather than generating your own.
Second, if a company wants a generative model trained on its own content, it should fine tune an existing model rather than starting from scratch. Fine-tuning and prompt training on specific content domains consume much less energy than training new large models, as well as offering better value than generically-trained models.
The third tip is to use less computationally expensive approaches such as TinyML to process the data. While general CPUs consume an average of 70 watts of power and GPUs consume 400 watts, a tiny microcontroller consumes just a few hundred microwatts to process locally.
Fourth, only use a large model when it offers significant value. Power-hungry models don’t necessarily result in significant gains in accuracy.
Fifth, depending on the context, it is not always necessary to use generative AI so be discerning. For example, generating a blog post doesn’t require a computation heavy tool, whereas predicting natural hazards does.
Sixth, evaluate the energy sources of your cloud provider or data centre to minimise carbon intensity. It is better to deploy models in regions that use environmentally friendly power resources.
Seventh, re-use models and resources. This means opting for open-source models rather than training new ones and recycling raw materials from laptops, processors and so on to make new ones.
Finally, AI activity should be included in the carbon monitoring carried out by AI research labs, vendors and the firms using AI, and the results publicised. Online tools like CodeCarbon can be used for monitoring and establishing benchmarks.
So what
Understanding what is involved in generative AI reveals the areas to target to make it greener. From the inception of the ideas for using generative AI to the infrastructure utilised to gain results, all need to be following green AI approaches.