Generative AI in Supply Chains: Applications and Challenges
Dr Timofey Shalpegin provides a thoughtful perspective on Generative AI in Supply Chains, in particular considering applications and challenges (including risk and sustainability) related to New Zealand industry.
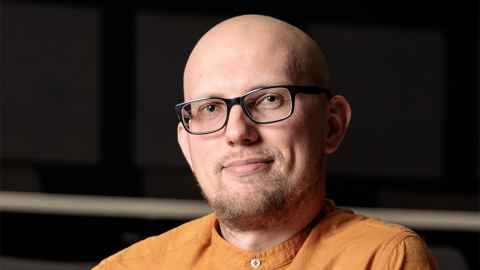
Likened by some to the invention of electricity, Generative AI (Gen-AI) has become a transformative force, pivotal to organisational success across industries – including New Zealand’s supply chain sector. Gen-AI generates new content, such as text, images, and data, by learning from vast amounts of input. Unlike traditional AI, which mainly focuses on task-specific optimisation, Gen-AI can create and innovate in ways previously limited to human expertise. This article overviews the breadth of its key applications and the implementation challenges it poses for supply chain management, informed by the latest research.
Among its most significant impacts, Gen-AI can democratise the use of traditional AI tools. Machine learning models, once used exclusively by highly skilled data scientists to forecast demand, manage inventory, and design supply chains, are now accessible to a broader range of supply chain professionals. This shift particularly matters as supply chains become more dynamic and complex. It lets those professionals interact with AI systems in natural language, simplifying tasks like introducing new constraints or adjusting parameters in fast-changing environments. Easier interaction further enhances machine learning capabilities.
In New Zealand, a significant impact is expected on agricultural supply chains. Deloitte predicts that Gen-AI, along with machine learning and neural networks, could help double the value of New Zealand’s agricultural exports. Key applications include optimising resource use and improving traceability, predicting disease outbreaks, and determining the optimal times for planting and harvesting. Gen-AI can also enhance animal disease diagnosis, improve resilience in farming operations, and propel advancements in precision agriculture, letting farmers make data-driven decisions that increase efficiency and sustainability. The red meat co-operative Alliance Group has already implemented AI-powered technology to assess and improve the eating quality of beef and lamb. Similar applications are being developed in the apple, wine, and other agricultural sectors.
While Gen-AI offers numerous benefits, it has been heavily criticised for its environmental impact, particularly due to the energy consumption required to train and maintain AI models. Developing Gen-AI systems involves processing vast amounts of data through complex algorithms, which demands significant computational power. Often the electricity comes from non-renewable energy, deepening its carbon footprint. Experts estimate that Gen-AI could consume around 1.5% of global energy by 2029. To put that in perspective: New Zealand currently consumes about 0.15% of world energy.
Yet, Gen-AI could compensate for its environmental footprint by positive contributions to supply chain sustainability. For instance, it can optimise operations, reducing waste and improving resource efficiency. Refined logistics and distribution routes help companies lower emissions, reduce unnecessary travel, and avoid delays. Additionally, easier traceability improves monitoring and reporting of sustainability metrics, which supports organisations in meeting their environmental targets and making their supply chains more eco-friendly overall. While likely to be positive on balance, Gen-AI’s impacts on sustainability may vary across regions. In areas heavily dependent on non-renewable energy, increased computational demands could cause a net negative environmental impact, whereas regions with access to renewable energy sources may experience happier outcomes.
Another promising application is risk management: Gen-AI enhances detection, assessment, and mitigation of risks. AI-driven models can simulate scenarios, including natural disasters. Gen-AI’s real-time monitoring, predictions and early warnings are particularly valuable in forestalling cyber threats.
One major challenge in implementing Gen-AI is its perception as a "black box": these systems often make decisions in ways that are difficult to interpret or explain. This opacity can undermine trust in AI-generated conclusions, especially for supply chain professionals who prioritise transparency and accuracy in decision-making processes. My fellow researchers and I have observed similar wariness among students using Gen-AI models in supply chain simulations. Even though the models are often trained on data relevant to the simulations and tend to offer (invisibly) well-reasoned suggestions, many students disregard the AI’s recommendations simply because they do not know, or therefore fully trust, the model’s reasoning.
Building trust in Gen-AI involves enhancing the interpretability and explainability of AI models. Efforts are underway to develop more transparent AI systems, often through techniques such as model-agnostic explainability methods. These methods clarify how AI systems reach their decisions. Ensuring that supply chain professionals can understand, validate, and trust AI-driven insights is crucial to successfully adopting Gen-AI in this field.
We must also beware ethical concerns around privacy, security, bias, and marginalising human judgement. Gen-AI systems often need access to large amounts of sensitive data. Discriminatory biases may sneak in, embedded in AI training data. And overreliance on AI in decision-making can sideline human intuition, creativity, and ethical considerations. Clear regulations and thoughtful integration of AI are imperative.
With its huge range of applications, Gen-AI is not just revolutionising today’s supply chains; it is laying the groundwork for those of tomorrow. Addressing challenges such as trust, transparency, and ethical concerns will be essential to fully harness its potential. As Gen-AI continues to evolve, supply chain professionals must remain adaptable, embracing these technologies so as to thrive in a rapidly changing landscape.
Dr Timofey Shalpegin is a Lecturer in Information Systems and Operations Management at the University of Auckland. t.shalpegin@auckland.ac.nz