AI can help address inequity – if companies earn users' trust
Considering the needs of the user and earning their trust is crucial to help address issues of AI inequity.
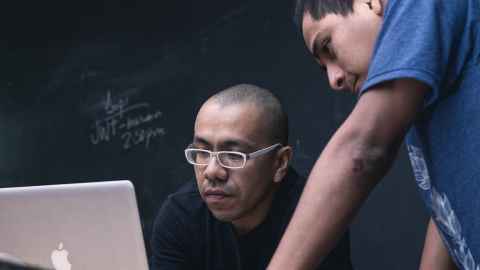
Article originally appeared on the Harvard Business Review website, 17 September 2021
By Shunyuan Zhang, Kannan Srinivasan, Param Vir Singh and Nitin Mehta
What/Focus
AI is making an ever-growing contribution to the global economy with AI algorithms providing numerous benefits for businesses and their customers. However, there is potential for AI inequity when algorithmic bias produces discriminatory outcomes for certain groups, typically minorities and women. One example is the recidivism prediction algorithms used in courts. However, the implications of AI inequity cut both ways in terms of receptivity to and adoption of AI. This article discusses the importance of earning users’ trust in AI to addressing inequity.
How (Details/Methods)
The authors studied an AI algorithm-based smart pricing tool launched by Airbnb that automatically adjusts a listing’s daily price according to various parameters of market conditions. While the tool increased revenue for those using it, it also increased the racial revenue gap as black hosts were significantly less likely to adopt it. The algorithm had tested well, but the reality was different.
It was concluded that firms must consider the following market conditions during AI algorithm creation: 1) the targeted users’ receptivity to an AI algorithm, 2) consumers’ reactions to algorithmic predictions, and 3) whether the algorithm should be regulated to address racial and economic inequalities by incorporating firms’ strategic behaviour in developing the algorithm.
First, with regard to receptivity, Black hosts were 41 percent less likely to adopt the algorithm, highlighting the need for inducements to encourage Black hosts to try the algorithm as well as sharing evidence of its benefits and how it works. Low SES was a factor, with education and income significant barriers to technology adoption. Building trust is therefore important in light of past racial bias in algorithms. Companies also need to provide evidence and explanations of the accuracy of algorithms. In sum, firms need to customise their algorithm promotion efforts to address these concerns.
The second aspect is recognising that how consumers react to AI decisions will shape the effect of the algorithm on market outcomes. With the Airbnb algorithm for example, although its adoption could combat racial inequity, black hosts were less represented in the data used to train the algorithm and another factor was the greater responsiveness found to price reductions for black-owned properties. Thus the market conditions also need to be factored in. Black hosts represent only nine percent of Airbnb properties, whereas white hosts represent 80 percent, meaning different optimal prices for black versus white hosts.
So What
The third aspect, or so what, concerns the strategic behaviour of decision-makers at the corporate or government level, who need to consider how the algorithm will be perceived by the targeted users. Underrepresented users may feel the algorithm is biased against them and users in general need to be aware of how the algorithm works. The second focus should be building trust through explaining what the algorithm is meant to do and how it works. Incentivising reluctant users is another important strategy.
For business to best combat algorithmic bias issues, considering the perception and adoption of algorithms and market conditions such as the ones described should be a major part of rolling out algorithmic tools.