Shipping operations management: past, present and future
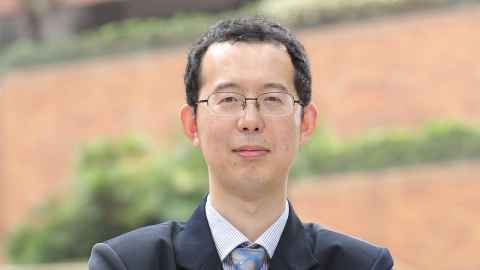
Shipping is the backbone of international trade with around 80 percent of global trade by weight fulfilled by maritime transportation. Shipping operations can be classified into tramp and liner. A ship operating in the tramp mode (usually tankers and dry bulk ships) transports a full shipload of cargo from one origin to one destination.
A ship operating in the liner mode (usually container ships) visits many ports according to a fixed sequence and schedule and transports cargo with many different origins and destinations simultaneously. In plain words, tramp shipping is similar to taxis, while liner shipping is similar to buses. Compared with land transport and air transport, shipping is less visible to academics because we see trucks in our cities and take flights but seldom have the experience of knowing how a ship operates.
Traditionally, shipping management studies employed methods including questionnaires and interview surveys (to determine key factors in port productivity), statistical analysis (to predict port cargo volumes based on historical throughput data and GDP) and network analysis (to compare and evaluate the connectivity of different ports based on existing shipping routes).
However, in the past two decades, researchers with broader educational backgrounds (industrial engineering, operations research, computer science) have worked on shipping management studies using new scientific tools, including mathematical optimisation, game theory and machine learning, as detailed below.
Mathematical optimisation
A basic question in liner shipping is how to design a liner shipping network, consisting of many liner shipping routes, to transport cargo at the lowest cost. This problem is similar to the vehicle routing problem (VRP) in operations research. A key difference is that, in a liner shipping network, cargo can be transferred between ships (similar to passenger transfer between two buses). This difference makes the liner shipping network design problem even harder than the VRP. Many businesses have employed methods such as branch-and-price, branch-and-cut, and large neighbourhood search to address this problem.
Game theory
In shipping, both the government and the shipping industry aim to provide efficient and reliable shipping services to ensure the smooth flow of trade. However, in terms of air emissions, their objectives differ.
The government designs regulations that control the air emissions from ships, and shipping companies react by adjusting their operations to minimise costs while meeting these requirements. For example, when the government specifies areas where ships must consume cleaner but more expensive fuel, shipping companies will reduce their speed in these areas to lower fuel consumption then sail faster in other areas to compensate for the time lost. Therefore, when a government designs a regulation, it must consider how the industry will react to it, rather than assume the industry will operate in the same way as before.
To characterise the interactions between government and industry, Stackelberg game models are applied by academics, in which the government is the leader and the industry is the follower.
Machine learning
A maritime authority is empowered to inspect foreign-flagged ships that visit the authority's ports to ensure they meet international maritime regulations and local laws. Due to cost considerations, only a small proportion (say 5 percent) of ships will be inspected. Using historical inspection records, machine learning models have been developed to predict a ship's condition based on factors such as age, type, tonnage, last inspection time, and historical condition, so ships in the worst condition are selected for inspection. The development of machine learning models should incorporate shipping domain knowledge. For example, an older ship is expected to have a worse condition than a newer one (in a statistical sense) when all the other factors are the same. Such monotonicity should be captured by the machine learning models.
The shipping industry continues to embrace new sciences, technologies, and policies. Looking ahead, some emerging topics in shipping operations management are autonomous ships, blockchain applications, low-carbon shipping, digitisation and drone applications.
Shuaian Wang is an Associate Professor at the Department of Logistics and Maritime Studies at Hong Kong Polytechnic University and is currently on sabbatical in the Department of Information Systems and Operations Management. His research interests include shipping network design, port operations planning, urban transport network modeling, sustainable transportation and green logistics, and supply chain management.